As you know, HDInsight is powerful service to analyze, manage and process BigData on Microsoft Azure. You can create Hadoop, Storm, Spark and other clusters pretty easily! In this article, I will introduce how to create Hive tables via Ambari with cvs files stored in Azure Storage.
At first, you have to create your HDInsight cluster associated an Azure Storage account. In this article, I create a Spark 2.1.x cluster as HDInsight cluster.
Store CSV files into your Azure Storage
Upload your CSV files into the Azure Storage account. In this article, I upload Nikkei Average CSV file like below.
DATE,CLOSE,START,HIGH,LOW
2012/1/5,8488.71,8515.66,8519.16,8481.83
2012/1/6,8390.35,8488.98,8488.98,8349.33
2012/1/10,8422.26,8422.99,8450.59,8405.18
2012/1/11,8447.88,8440.96,8463.72,8426.03
2012/1/12,8385.59,8423.1,8426.83,8360.33
2012/1/13,8500.02,8471.1,8509.76,8458.68
2012/1/16,8378.36,8409.79,8409.79,8352.23
2012/1/17,8466.4,8420.12,8475.66,8413.22
2012/1/18,8550.58,8458.29,8595.78,8446.09
2012/1/19,8639.68,8596.68,8668.94,8596.68
2012/1/20,8766.36,8751.18,8791.39,8725.32
2012/1/23,8765.9,8753.91,8795.27,8744.54
2012/1/24,8785.33,8815.36,8825.09,8768.51
2012/1/25,8883.69,8842.01,8911.62,8816.09
2012/1/26,8849.47,8890.49,8894.6,8834.93
2012/1/27,8841.22,8851.02,8886.02,8810.89
2012/1/30,8793.05,8803.79,8832.48,8774.23
2012/1/31,8802.51,8781.44,8836.68,8776.65
It's stored into Azure Storage account associated with HDInsight cluster, and its path is described as "https://"storage-account-name"."spark-container-name".blob.core.windows.net/financedata/nikkei_stock_average_daily_en.csv". You can specify the path in Hive query as "wasb://"spark-container-name"@"storage-account-name".blob.core.windows.net/financedata/nikkei_stock_average_daily_en.csv".
Create Hive tables from your CSV files
Open https://portal.azure.com/ and choose your HDInsight cluster. You can open Ambari portal to click a link of "https://'your-cluster-name'.azurehdinsight.net" in "Overview" page. Next, click "Hive View 2.0" button like below.
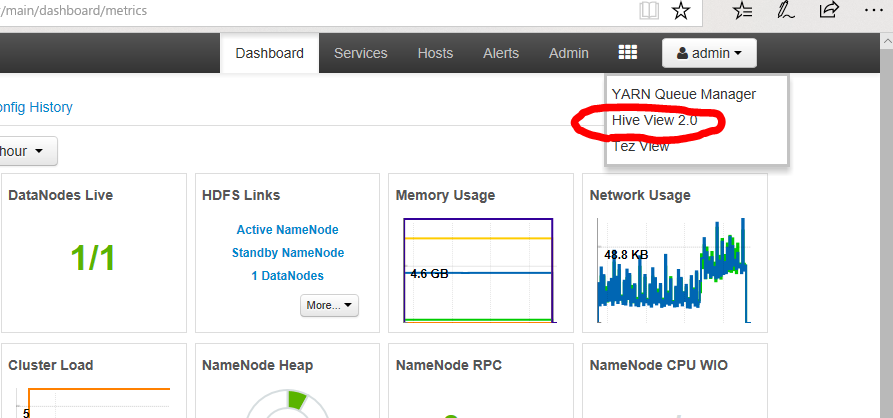
Now, you can execute Hive query using below portal site.
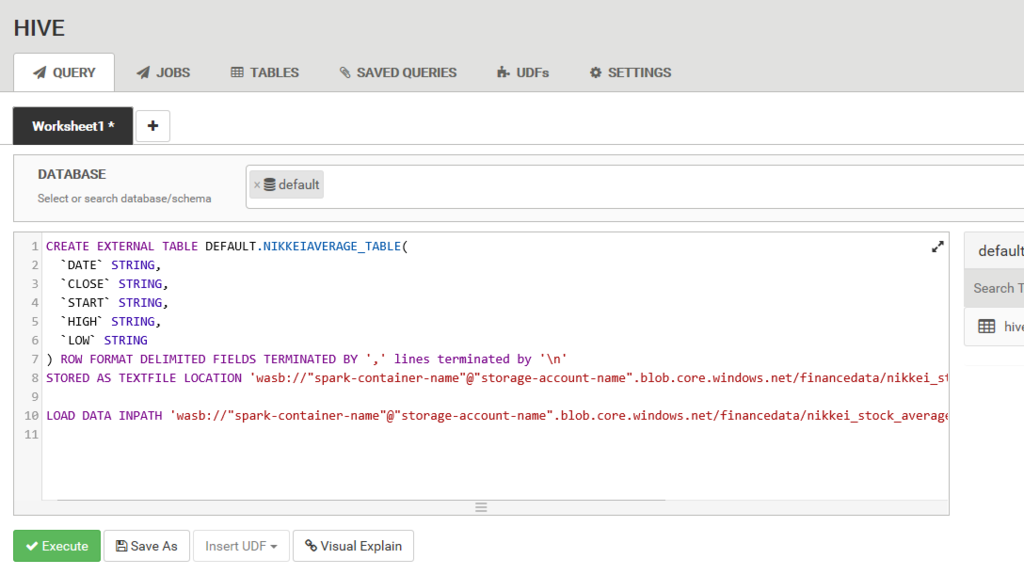
Copy below query and execute it into the site.
CREATE EXTERNAL TABLE DEFAULT.NIKKEIAVERAGE_TABLE(
`DATE` STRING,
`CLOSE` STRING,
`START` STRING,
`HIGH` STRING,
`LOW` STRING
) ROW FORMAT DELIMITED FIELDS TERMINATED BY ',' lines terminated by '\n'
STORED AS TEXTFILE LOCATION 'wasb://"spark-container-name"@"storage-account-name".blob.core.windows.net/financedata/nikkei_stock_average_daily_en.csv';
LOAD DATA INPATH 'wasb://"spark-container-name"@"storage-account-name".blob.core.windows.net/financedata/nikkei_stock_average_daily_en.csv' INTO TABLE DEFAULT.NIKKEIAVERAGE_TABLE;
Check your query result
After executing your query, run below query to check the data in Ambari.
SELECT * FROM DEFAULT.NIKKEIAVERAGE_TABLE;
So, you can get below result.
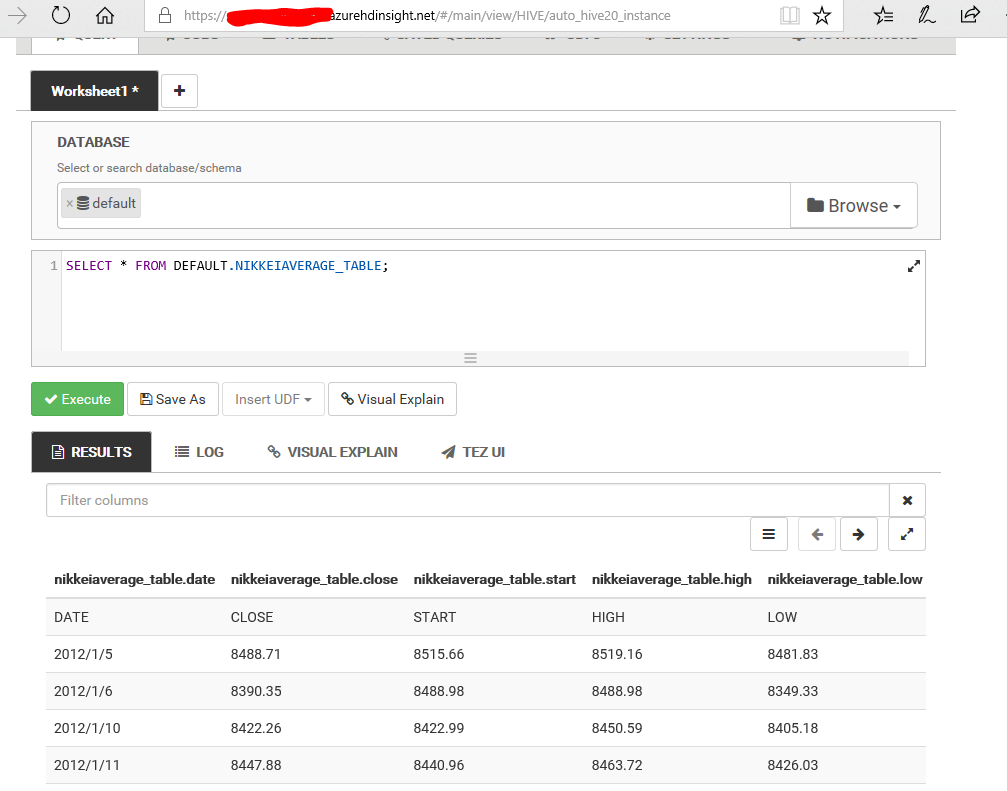